In today’s rapidly advancing technological landscape, many industries now depend heavily on machine learning, impacting decision-making processes and shaping our daily lives. However, with this increasing reliance on machine learning systems comes the need for ethical considerations to ensure fairness, transparency, and responsibility.
This article aims to explore the concept of Ethical Machine Learning, examining the ethical considerations, best practices, and challenges associated with this evolving field. But before that, it is important to understand what machine learning is and why ethical machine learning is important.
- RELATED – Operationalizing Machine Learning in Processes
- Mixed reality Vs Augmented Reality Vs Virtual reality
What is Machine Learning?
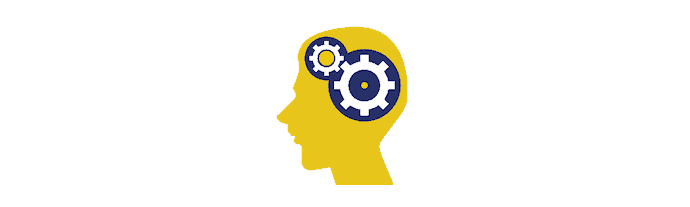
Machine Learning is a branch of artificial intelligence that enables computers to learn and make predictions or decisions based on data. It involves developing algorithms and models that can identify patterns, extract insights, and improve their performance over time without explicit programming.
Machine Learning algorithms learn from labeled or unlabeled data, allowing them to recognize patterns and relationships. There are different types of Machine Learning, including supervised learning (using labeled data for training) and unsupervised learning (finding patterns in unlabeled data). Machine Learning has diverse applications in various fields, such as image and speech recognition, natural language processing, recommendation systems, and fraud detection, which you can learn in Machine Learning courses. Its ability to process vast amounts of data and make accurate predictions makes it a powerful tool for solving complex problems and driving innovation across industries.
Ethical Considerations in Machine Learning
Machine learning algorithms have the potential to perpetuate bias, discrimination, and unfairness. Understanding these ethical considerations is crucial to address such issues and ensuring equitable outcomes. One of the main challenges is bias and fairness, as algorithms can unknowingly perpetuate biases present in training data, resulting in unfair outcomes. To mitigate this, it is essential to identify and address bias in the data and algorithms, ensuring fairness and equity in machine learning systems.
Another critical ethical consideration is privacy and data protection. As machine learning systems rely on vast amounts of data, concerns arise regarding the collection, storage, and usage of personal information. Ethical practices involve adopting data anonymization techniques, implementing strong data protection measures, and ensuring compliance with relevant data privacy regulations to safeguard individuals’ privacy rights.
Transparency and explainability are also fundamental in ethical machine learning. The opaqueness of machine learning algorithms poses challenges in understanding how they arrive at decisions. To establish trust and accountability, it is crucial to develop transparent machine-learning models that can be interpreted and explained. This promotes transparency, allowing users to understand the rationale behind algorithmic decisions and detect any potential biases or errors.
Accountability and responsibility lie at the core of ethical machine learning. Developers and organizations have an ethical responsibility to design, develop, and deploy machine learning systems responsibly. This includes ongoing monitoring to identify and address algorithmic biases and errors. Ethical decision-making frameworks provide guidance for the responsible use of machine learning, ensuring accountability and mitigating any potential negative impact on individuals or society.
Ethical Machine Learning Practices
Ethical machine learning practices encompass several key areas. Ethical data collection and handling are essential to maintain the integrity and privacy of data. This involves ethical considerations in data collection, such as obtaining informed consent, ensuring data accuracy, and implementing proper data governance and management practices.
Model development and deployment also require careful ethical considerations.
Developers should strive for fairness and avoid bias during the model development phase. Regular model audits and performance evaluations are necessary to identify and mitigate any biases or errors that may arise. Ethical implications during model deployment, such as considering the impact on different user groups and ensuring responsible deployment, are crucial for the ethical use of machine learning.
Adherence to ethical guidelines and regulations is vital in promoting responsible machine learning practices. Several organizations and institutions have established ethical frameworks and guidelines that outline best practices for the development and deployment of machine learning systems. It is essential for organizations to adopt these guidelines and comply with relevant regulations to ensure ethical behavior and accountability.
Why Is It Important To Consider Ethical Machine Learning?
Considering the ethical aspects of Machine Learning is crucial for several reasons. It ensures fairness and mitigates bias by identifying and addressing biases present in the training data. Ethical considerations promote equitable outcomes and prevent discrimination.
Ethical Machine Learning promotes trust and transparency by enabling users to understand how decisions are made. It fosters accountability and responsible behavior among developers and organizations. Ethical guidelines also protect privacy rights and ensure compliance with relevant regulations.
Future Trends and Challenges in Ethical Machine Learning
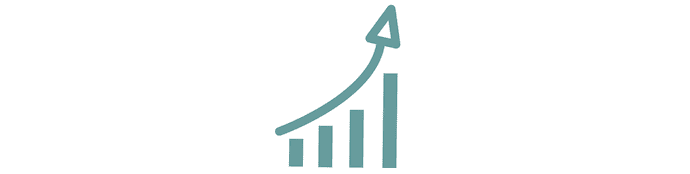
Ethical machine learning is an evolving field with emerging trends and challenges. As new technologies such as deep learning, reinforcement learning, and automated decision-making systems gain prominence, it becomes crucial to assess their ethical implications. These technologies often introduce complex ethical challenges, such as interpretability, fairness, and human oversight. Continued research, collaboration, and ethical discussions are needed to address these challenges and ensure the responsible development and deployment of machine learning systems.
Automated decision-making systems based on machine learning algorithms are becoming more prevalent in various domains. Ethical considerations surrounding these systems include fairness, transparency, and accountability. Ensuring that automated decision-making systems align with ethical principles is vital to avoid potential biases and ensure that individuals’ rights and well-being are protected.
Addressing ethical challenges in AI and machine learning is an ongoing effort. As technology evolves, new ethical dilemmas emerge. Striking a balance between innovation and responsible use is crucial. Organizations and researchers must collaborate to address the ethical challenges posed by AI and machine learning, promoting transparency, accountability, and societal benefit.
Wrapping Up
Ethical Machine Learning is of paramount importance in today’s AI-driven world. As machine learning systems continue to shape our lives, it is essential to ensure fairness, transparency, and responsibility in their development and deployment. Ethical considerations, such as bias and fairness, privacy and data protection, transparency and explainability, and accountability and responsibility, play a pivotal role in promoting ethical practices.
By addressing bias in data and algorithms, implementing privacy measures, fostering transparency, and adopting ethical guidelines and regulations, we can harness the potential of machine learning while safeguarding against potential pitfalls. Ethical machine learning practices empower individuals, build trust in AI systems, and help prevent harmful consequences.
It is crucial to remain vigilant and adopt ethical practices to emerging trends and challenges. Continued research, collaboration, and engagement among academia, Masters in Machine Learning, industry, policymakers, and society at large are vital in shaping a future where machine learning systems align with ethical principles and contribute positively to our world.
Ethical Machine Learning is not a mere requirement but an ethical imperative. By prioritizing ethics in machine learning, we can foster a responsible and trustworthy ecosystem that empowers individuals, safeguards their rights, and ensures that AI-driven technologies serve the greater good of humanity.
I hope this tutorial helped you to know about “What is Ethical Machine Learning?“. If you want to say anything, let us know through the comment sections. If you like this article, please share it and follow WhatVwant on Facebook, Twitter, and YouTube for more Technical tips.
What is Ethical Machine Learning – FAQs
What is Ethical Machine Learning?
Fairness in Machine Learning refers to the idea that the model’s predictions should be unfairly biased against certain groups of people.
What is meant by the Ethical approach in AI?
AI ethics is a set of guidelines that advise on the design and outcomes of artificial intelligence.
Why is ethical learning important?
It helps categorize discipline, honesty, and integrity in our actions performed during the day. Acting as a guideline to influence behavior and make the right choice, it is easier for individuals to abide by rules and act responsibly with ethics. Ethics in education helps run the system smoothly.
What exactly is Machine Learning?
Machine Learning is an AI technique that teaches computers to learn from experience. Machine Learning algorithms use computational methods to “learn” information directly from data without relying on a predetermined equation as a model.
What are the types of Machine Learning?
There are primarily three types of Machine Learning: Supervised, unsupervised, and Reinforcement Learning.